Current projects:
Myocardial blood flow quantification with 82Rb PET
At the University of Ottawa Heart Institute, National Cardiac PET Centre we have been developing PET for routine quantification of myocardial blood flow (MBF) and myocardial flow reserve (MFR). These efforts have resulted in the development and commercialization of the following technologies:
Our current efforts are focused on improving precision of MBF and MFR by tackling problems such as respiratory and patient motion correction.
Collaborators:
- Robert. deKemp, PhD, University of Ottawa Heart Institute, Cardiac PET Centre
- T. Xu, PhD, Carleton University, Department of Physics
- A Alessio, PhD, Michigan State University, Department of Computational Mathematics, Science, and Engineering; Biomedical Engineering and Radiology; Institute for Quantitative Health Science & Engineering
Students:
- Spencer Manwell, PhD, Department of Physics, Carleton University, Thesis
- Chad Hunter, Postdoctoral Fellow
- Anbhu Sritharan, MASc, Department of Systems and Computer Engineering, Carleton University, Thesis
- Simisani Takobana, MASc, Department of Systems and Computer Engineering, Carleton University, Thesis
Lesion synthesis in FDG PET and CT
We have developed state-of-the-art methods for synthesizing realistic lesions in both PET and CT data. We are currently applying these methods to generate large libraries of images with well characterized fake lesions for the following goals:
- Characterize the limits of detection (LOD), including lesion contrast, lesion size, image noise and anatomical region, of human-observers under fixed imaging conditions such as PET image reconstruction method using synthetic lesions.
- Optimization of PET image reconstruction and visualization for lesion detection on the merits of LOD.
- Developing machine-learning based lesion detection artificial intelligence (AI) using very large libraries of synthetic lesions.
- Objectively comparing the performance of clinicians and AI for the task of lesion detection with regards to both success rates and LOD.
Collaborators: Charles Collin, Elizabeth Krupinski, General Electric Health Care PET R&D team,
Student:
- Hanif Gabrani-Juma, MASc, Biomedical Engineering, Carleton University, Thesis
- Quinn de Bourbon, MSc, Medical Physics, Carleton University, Thesis
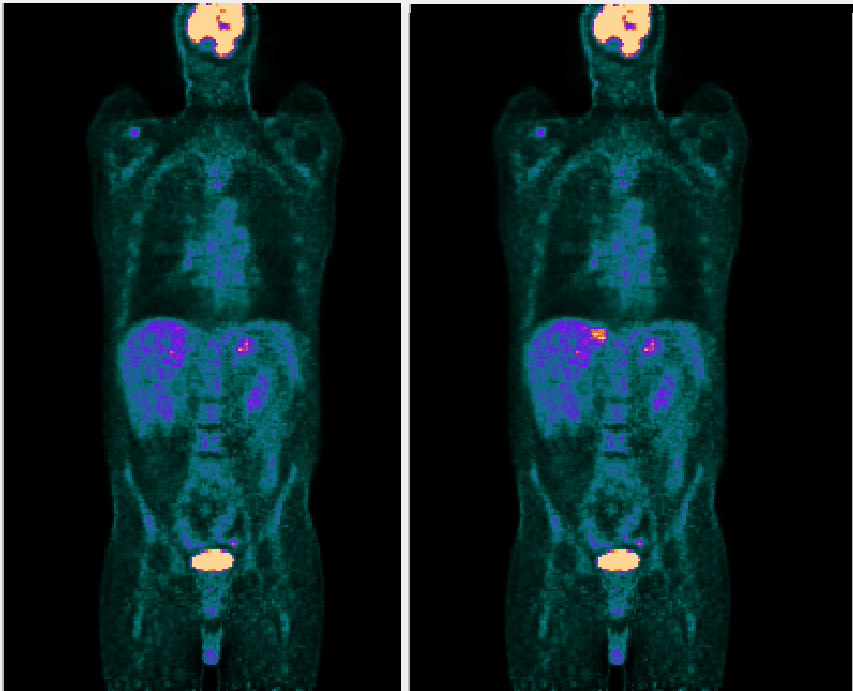
Enhancement, Segmentation, and Classification of Lung Ventilation and Perfusion Scintigraphy
In collaboration with Dr Eric Moulton of Jubilant-Radiopharma, we are developing AI for interpretation of lung V/Q studies.
Students:
- Siraj Ghassel, MASc, Computer Science, University of Ottawa, Thesis
- Amir Jabbarpour, PhD Candidate, Medical Physics, Carleton University
Prediction of Kidney Failure and Optimal Therapy
These collaborative efforts include amongst others nephrologists
Ayub Akbari and
Gregory Hundemer, Biochemist
Chris McCudden and Engineering Professor
James Green. This group is developing predictive algorithms for timely selection of patients with chronic kidney disease in the Multicare Kidney Clinic that are likely to require renal replacement therapy in the near-future. Furthermore, we are endeavoring to develop algorithms to guide clinicians for optimal Tacrolimus dosing in renal transplant recipients.
Students:
- Martin Klamrowski, MASC, Electrical and Computer Engineering, Carleton University, Thesis; and PhD Candidate
- Elmira Amooei, PhD Candidate, Biomedical Engineering, Carleton University.
Recent Projects
FDG PET-CT Image segmentation and lesion detection
Using analytical methods and machine-learning we are developing artificial intelligence (AI) for computer aided diagnosis (CAD) of FDG PET-CT studies. In collaboration with Hermes Medical Solutions we are enabling collaboration between human and machine observers using Hybrid3DTM to improve diagnostic accuracy, automatically generate templated clinical reports, and to provide active learning feedback to our AI.
Past Student: Odai Salman, PhD, Systems and Computer Engineering, Carleton University, Thesis.
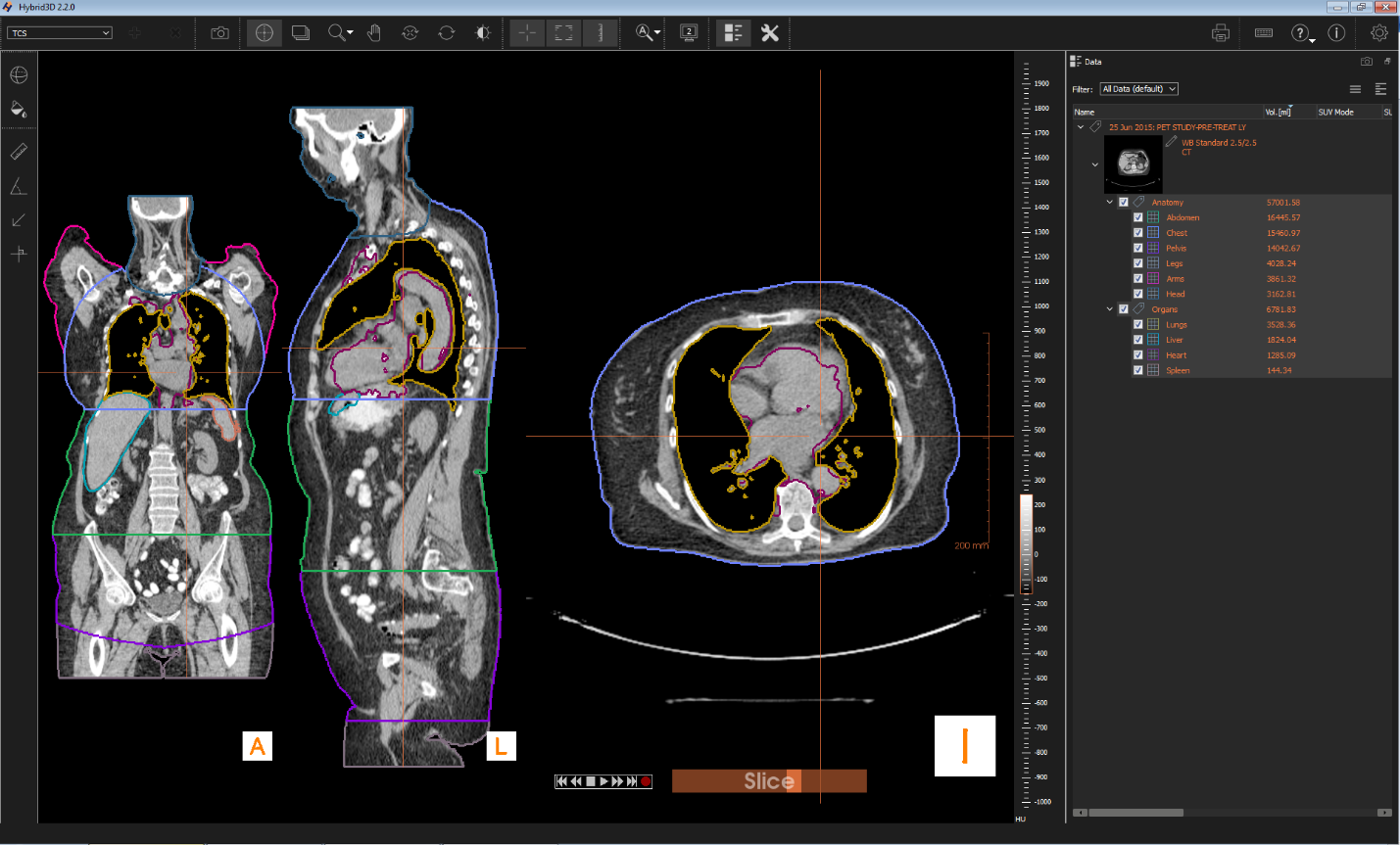
Two-compartment exchange phantom
With Catherine Coolens and Shelley Medical Solutions we have developed and validated a two-compartment exchange phantom for multimodality imaging. The
DCE perfusion from phantom phantom can be used for validation of quantitative dynamic imaging including PET, SPECT, CT and MRI for modality development, qualification testing in clinical trials, and quality control.
Collaborators: C. Coolens
Student: Hanif Gabrani-Juma, BSc, Department of Systems and Computer Engineering, Carleton University
Industry partner: Shelley Medical Imaging Technologies
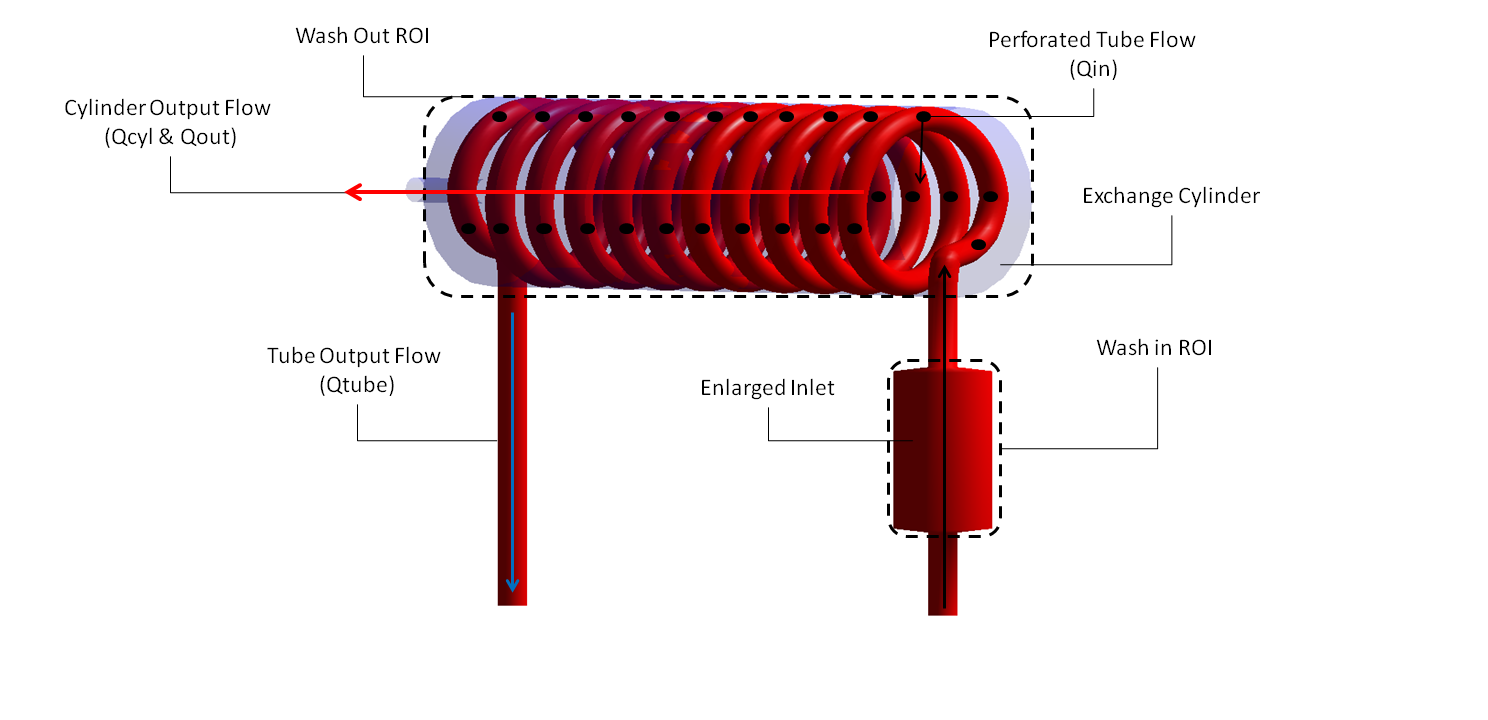
Quantitative SUV SPECT
Quantitative SPECT that can generate SUV scaled images has become routinely available, but its clinical utility is not yet clear. Since 2015 we have been increasingly applying SUV SPECT imaging in routine clinical practice to enable research of its clinical utility. To date we have produced numerous publication and abstracts using SUV SPECT and parathyroid adenomas, thyroid imaging and tumor imaging.
Collaborators:
L. Zuckier, W. Zeng
Industry partner: Hermes Medical Solutions